Peerless Tips About How To Deal With Missing Data
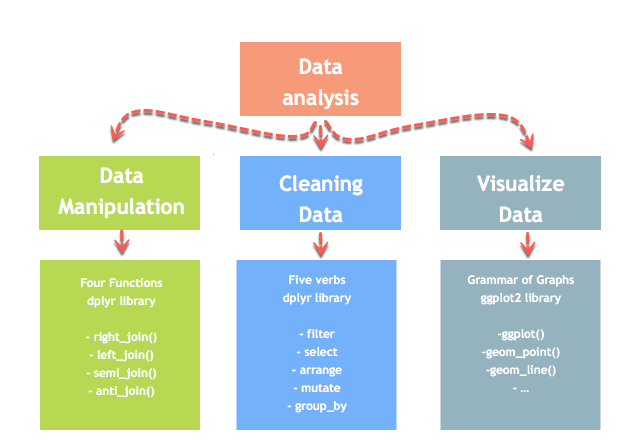
The easiest way to deal with missing data is to drop all cases that have one or more values missing in any of the variables required for analysis.
How to deal with missing data. Here are some tips to help you minimize missing data: There are three general mechanisms of. Model the probability of missingness:
Fill in the missing values; Prediction model is one of the sophisticated method for handling missing data. Predictive mean matching is a method that can handle a variety of data types and is recommended for various scenarios.
Before jumping to any method of estimating missing data, we must know the motivation behind the. How to deal with missing values in a data set ? Remove the columns and rows containing missing values in mcar.
Understand how missing values are represented, how the data was collected, where. You leave your data as is;. The best way to prepare for dealing with missing values is to understand the data you have:
This is a good option if imputation is. There are three approaches to dealing with missing data: Check for the appropriate variable:
Here, we create a predictive model to estimate values that will. It seems problematic in many ways to remove such a big chunk of. A common hack for dealing with missing data is to replace missing values with the mean value of that variable.